Computational Neuroscience(ATR International)
Research Staff
-
Professor
Motoaki KAWANABE -
Associate Professor
Norikazu SUGIMOTO
kawanabe@atr.jp |
|
To the site | https://bicr.atr.jp/cns-naist/en/ |
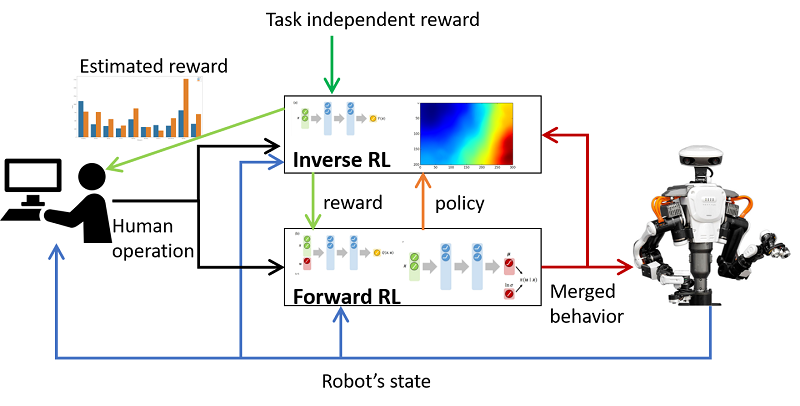
Fig.1 Machine intelligence for humanoid robot control
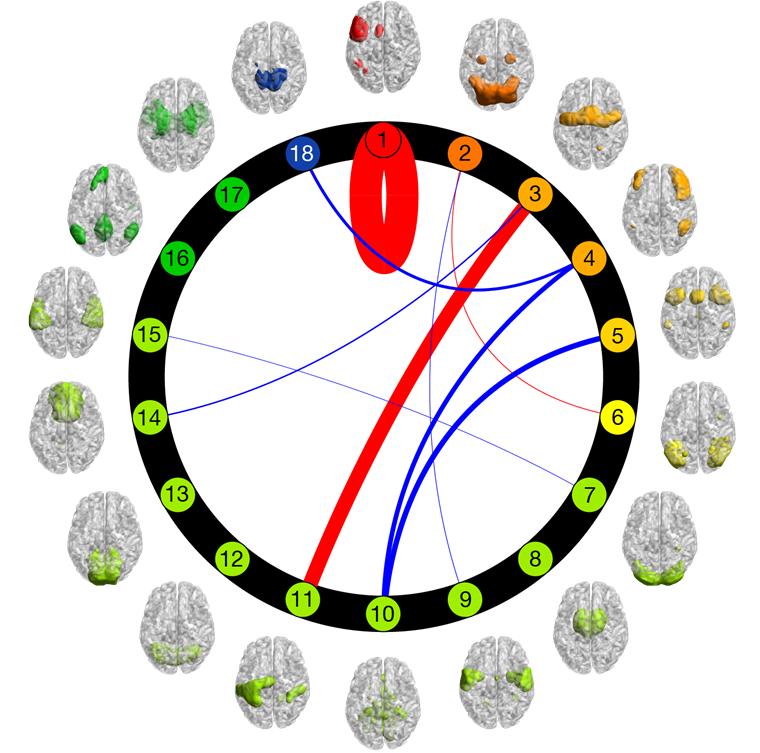
Fig.2 Brain network supporting a cognitive function (working memory)
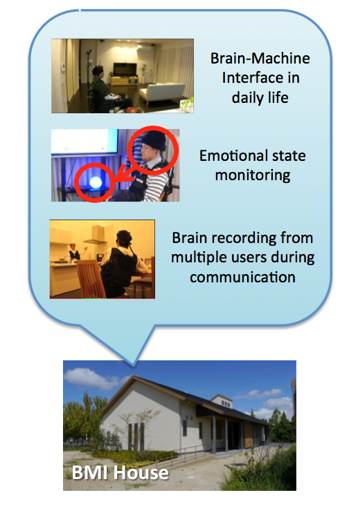
Fig.3 Brain-Machine Interface (BMI) in daily life
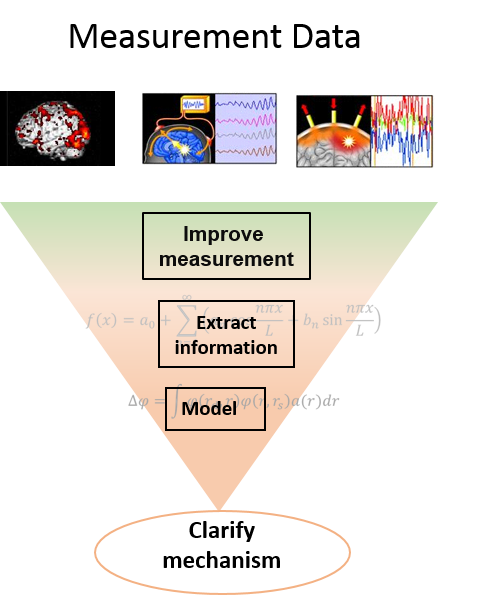
Fig.4 Multi-modal integration approach
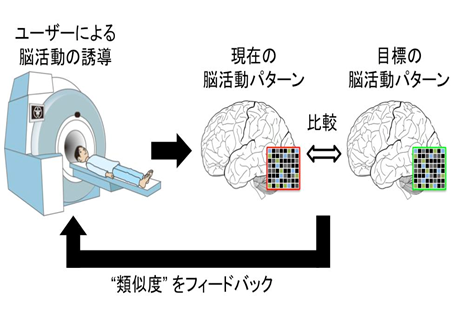
Fig.5 Neurofeedback
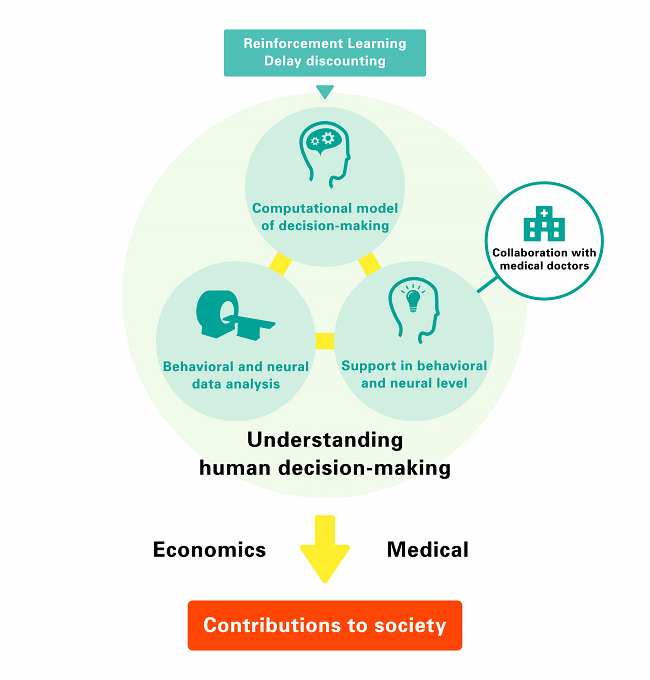
Fig.6 Computational model of decision-making
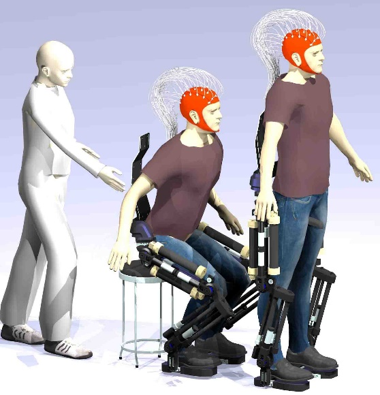
Fig.7 Adaptive shared control for BMI exoskeleton robots
Research Areas
We aim to understand the human brain and to achieve new machine intelligence (artificial intelligence) based on brain information processing functions. We conduct research and educate students on computational neuroscience and cutting-edge machine intelligence with such methodologies as brain decoding, brain machine interfaces, neurofeedback, and robot learning at ATR, an internationally recognized computational neuroscience center.
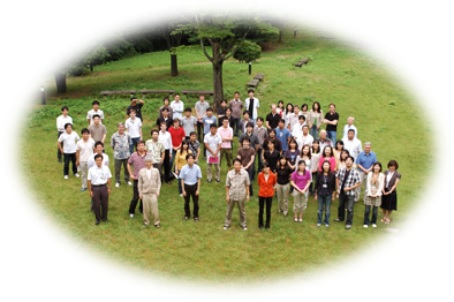
Key Features
Machine intelligence for humanoid robot control
How humans make decisions and control their own bodies can be formulated using reinforcement learning and control theory. Using data-driven reinforcement learning algorithms, we construct machine intelligence for human-in-the-loop robot control.
Cognitive functions: understanding and manipulation
The brain is a huge information network. We tackle enigmas in relationships between the brain network and cognitive functions such as memory and thinking. We develop neurofeedback techniques for preventing impairments to cognitive functions due to brain diseases and aging.
Brain-Machine Interface (BMI) in daily life
By measuring brain activities in daily living environments, we develop techniques to estimate mental states such as stress and empathy. Based on them, we approach the neural bases of cognitive functions in natural situations to pursue social applications of neuroscientific knowledge, including human resource development.
Novel analysis methodology development to understand brain functions
We aim to provide new ways to understand brain functions by developing innovative analysis methodology using statistical and machine learning theory. In particular we emphasize the multimodal data integration approach to overcome limitations of single measurement data.
Neurofeedback
We integrate psychophysical, neuroimaging, and computational neuroscientific approaches and propose novel neurofeedback methods, developing effective methods for BMI, medical treatment, and communication applications.
Computational models of decision-making
Our goal is to understand how humans make decisions. Reinforcement learning models and economic theorems allow us to build neural computations for human decision-making. We apply them to solve social, economic, and medical problems.
Adaptive shared control for BMI exoskeleton robots
Since robots are expected to work closely with humans, the development of a shared control strategy is becoming an increasingly important research direction. We are constructing an adaptive shared control strategy for our brain-machine-interface (BMI) exoskeleton robot.