Computing Architecture
Research Staff
-
Professor
Yasuhiko NAKASHIMA -
Affiliate Professor
Mutsumi KIMURA -
Affiliate Professor
Renyuan ZHANG -
Assistant Professor
KAN Yirong -
Assistant Professor
Hoai Luan PHAM -
Assistant Professor
Vu Trung Duong LE
nakashim@is.naist.jp rzhang@is.naist.jp kan.yirong@is.naist.jp pham.luan@is.naist.jp le.duong@naist.ac.jp |
|
To the site | http://archlab.naist.jp/ |
To the FACEBOOK | https://www.facebook.com/NAIST.ARCH |
Research Areas
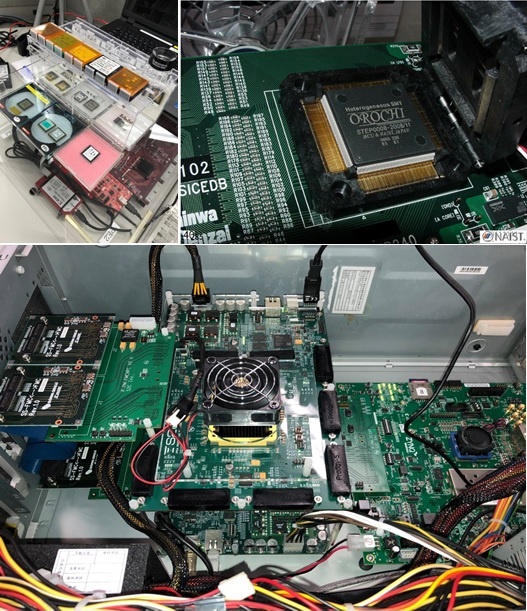
Fig.1: Power Efficient Near-Data Memory Array Accelerators and FPGA Systems
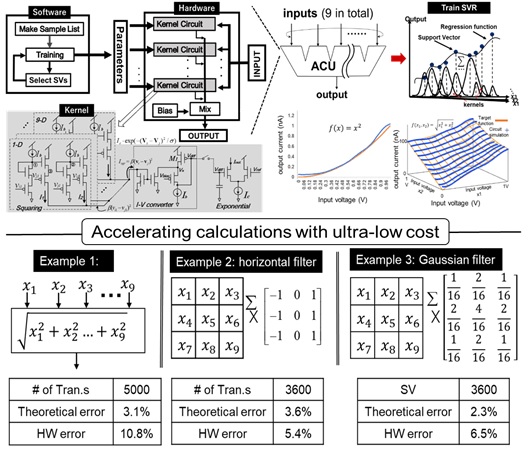
Fig.2: Analog Acelerators
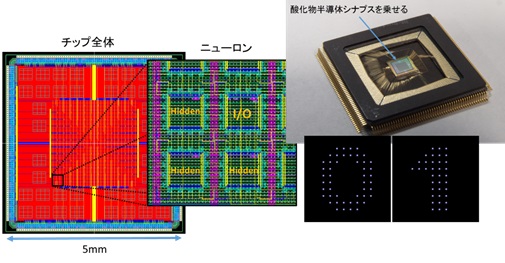
Fig.3: Analog Neural Network LSIs
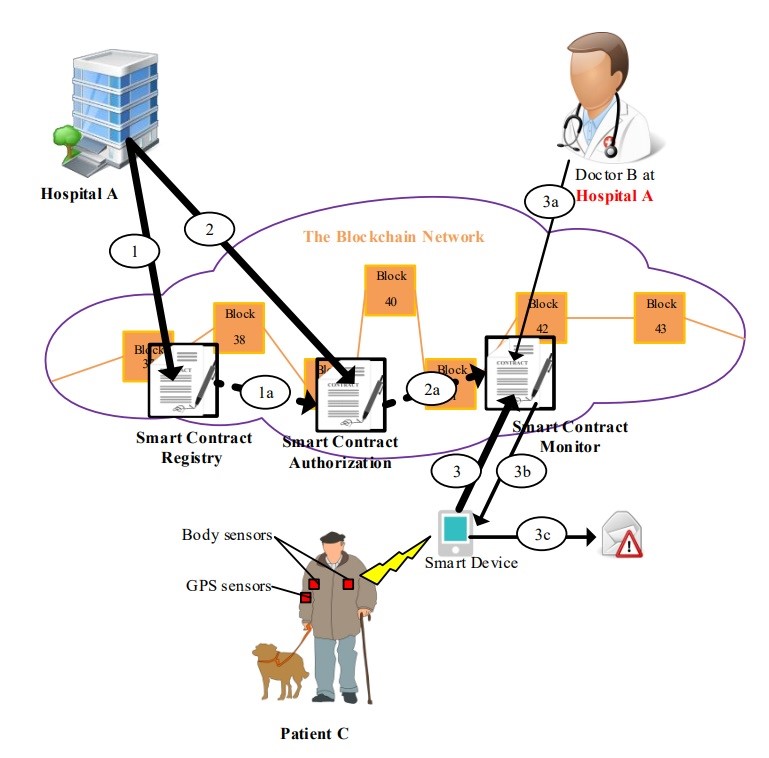
Fig.4: Blockchain for IoT
Power efficient near-data memory array accelerators for the Post-Moore Generation
Research and development of highly efficient computing systems, accelerators and LSIs for image processing and big data processing, such as graph processing and machine learning
- EMAXV,VR: A large-scale CGRA for image recognition
- IMAX: A small footprint near-memory accelerator for AI
Compact and efficient approximate computing VLSIs for the Post-Moore Generation
Research and development of reconfigurable approximate computing VLSI architectures with compact circuits, low energy, and function-flexibility for multi-operand computations, which can be efficiently employed in parallel computing tasks
- Various non-binary-based computing methodologies such as neuromorphic and stochastic computing for the Post-Moore generation
- Exploring analog-digital-hybrid CGRA platforms
Neuromorphic LSIs for the Post-Moore Generation
Research and development of super compact and low power neuromorphic integrated systems for artificial intelligence
- Amorpous metal-oxide semiconductor thin-film synapses for 3D structures
- Neuromorphic architecture and leaning rules for astronomical scale integration
- Brain-type integrated systems with artificial humanity
Energy efficient system architecture for next generation machine learning
Research and development of next generation machine learning system architecture with edge computing
- Probabilistic reasoning algorithms with Bayesian networks
IoT + blockchain for secure smart systems
IoT and blockchain technologies are combined to develop smart systems such as smart healthcare systems, smart city management, etc. Secure health monitoring systems for hospitals are currently being researched.
Key Features
In our laboratory, we study state-of-the-art technologies for next-generation computing paradigms. Our goal is to realize environment-friendly, high-performance, and robust computer systems under energy constraints. From a wide viewpoint (from new theories to LSI implementations), we promote cutting-edge research and the highest degree of education within various research themes, particularly: high-performance, low-energy and dependable computation, and hardware/software co-design.